Artificial intelligence (AI) offers tremendous potential for businesses, yet many organizations struggle to turn pilots into full-scale successes. Why? Often, it’s the unchecked risks, ethical concerns, or lack of stakeholder trust that cause AI initiatives to fail. According to recent McKinsey research, a staggering 90% of AI projects are stuck in the experimentation phase.1
What is AI Governance?
Enter AI governance: An essential framework of policies, processes, standards, and guardrails designed to ensure AI systems and tools are safe, ethical, and aligned with the right outcomes. A key component of a business’s AI strategy, AI governance frameworks direct AI research, development, and application to help ensure safety, fairness, and respect for human rights.
This post explores how intentional AI governance can accelerate innovation and outlines the essential components leaders need to build frameworks that unlock AI’s potential–safely and responsibly.
Why AI Governance Matters
When successful, AI Governance becomes a powerful enabler of innovation across the organization:
AI Governance as a Catalyst, not a barrier
It’s a common misconception that governance hinders innovation. Forward-thinking organizations see AI governance not as a barrier, but as the key to unlocking the safe and secure adoption that drives ROI. Clear ethics and risk management empower teams to pursue bold ideas without the worry of last-minute roadblocks or compliance failures.
Despite heavy investment in AI, many initiatives never make it to production due to concerns about bias, security, or compliance. Effective governance breaks this paralysis by building trust. It assures internal stakeholders and external regulators alike that AI systems are being developed responsibly, which in turn enhances trust in their outcomes. Internally, developers and business units gain confidence to push AI projects forward, knowing there’s a process to prevent major missteps.
A well-structured and aligned framework helps direct effort to high-impact use cases. By proactively rolling out governance initiatives, companies can accelerate AI deployments and unlock new efficiencies that may have eluded them.
Bottom line: AI governance acts as a catalyst, transforming experiments into responsible, repeatable innovation.
Alignment with Clear Outcomes
AI governance ties initiatives to clear business goals and establishes accountability. By reviewing initiatives and use cases for strategic fit, ROI, and impact, governance ensures resources focus on AI innovations that truly support the organization’s mission. This alignment also means efforts are more likely to deliver tangible value.
Oversight mechanisms and observability help track progress and keep projects on course. If a system isn’t performing as expected or causes unintended outcomes, the right processes prompt timely intervention. This structure helps ensure AI efforts remain purposeful and effective, enabling innovation that’s both sustainable and supported across the business.
Enhanced Trust and Reputation
Quality and trust are essential for AI adoption, and a strong governance program greatly builds that trust. Internally, teams trust outputs more when models have passed reviews for accuracy, fairness, bias, and security. Externally, customers, partners, and regulators gain confidence knowing the company has oversight of its AI initiatives.
Governance measures like transparency reports, bias audit results, and compliance certifications demonstrate that the organization is serious about responsible AI. This not only helps prevent regulatory issues, PR disasters, and fines, but also enhances the organization’s credibility and public trust.
Key Pillars of Effective AI Governance Frameworks
To enable responsible innovation, an AI governance framework should cover several key pillars. These establish the policies and processes needed to harness AI safely and effectively:
Intake and Prioritization
A clear intake process is crucial for aligning AI efforts with business strategy, technical viability, and ethical principles from the start. Governance should include a structured innovation process for submitting and evaluating new AI use cases. This intake typically captures problem statements, desired outcomes, data requirements, risk levels, and strategic alignment for consistent review and comparison.
Since not all use cases have equal value or risk, prioritization based on strategic fit, potential impact, and complexity is essential. High-impact, low-risk initiatives should be fast-tracked, while riskier or more complex ones need thorough discovery and safeguards. This ensures resources are focused on the most important initiatives, making governance a strategic enabler of responsible AI innovation.
Risk Management and Compliance
Effective AI governance starts with proactive risk management. Identifying and mitigating potential issues–such as data breaches, security vulnerabilities, bias, and regulatory non-compliance–early helps prevent problems before they derail a project. This requires embedding assessments and checkpoints throughout the AI lifecycle, from initial ideation and development to deployment, fine-tuning, and ongoing monitoring. Aligning with emerging regulations is just as critical. Staying ahead of legal requirements doesn’t just reduce risk–it builds credibility and gives leaders greater confidence in their AI initiatives.
High-risk use cases should face additional scrutiny, including bias audits, validation, and legal or compliance reviews before deployment. Defining what qualifies as low, medium, or high risk is equally important, ensuring teams apply the right level of oversight for each scenario. The figure below shows different risk categories and examples of how governance controls should scale accordingly.
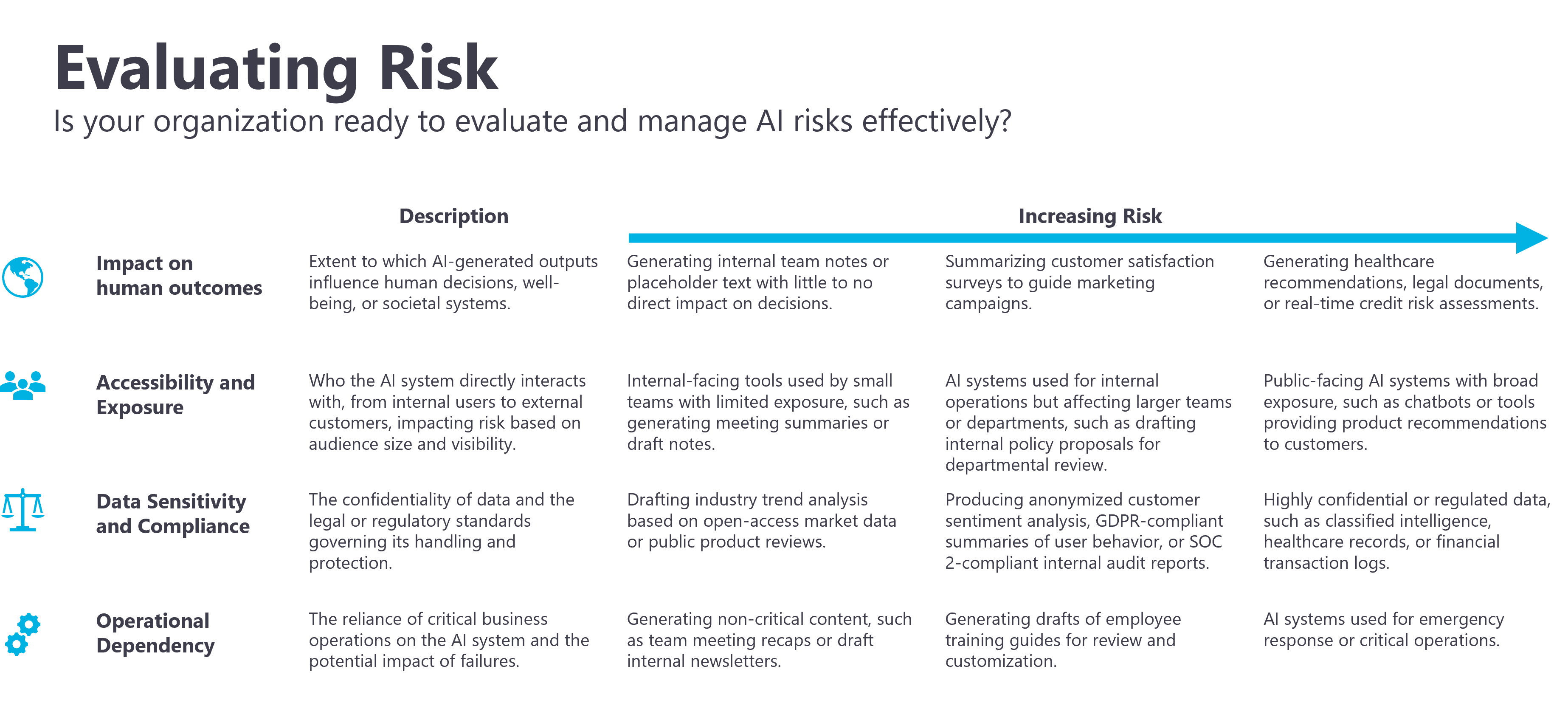
Ethical Oversight and Fairness
Generative AI presents unique ethical complexities that surpass traditional software and machine learning risks. To proactively address these, organizations should integrate explicit ethical principles into their governance frameworks. This goes beyond stated values and includes actionable commitments to:
- Actively mitigating bias and ensuring equitable outcomes across diverse groups or populations.
- Providing insights into how models arrive at outputs, particularly to identify and address potential sources of bias or unfairness.
- Establishing clear points of human review and intervention, especially in high-stakes use cases.
- Ensuring the AI is reliable and doesn’t produce outputs that could be unfairly harmful, discriminatory, or misleading.
Transparency & Accountability
Clear, traceable decision-making is fundamental to responsible AI. AI governance frameworks should create visibility into how AI systems operate, including the data they rely on, how models are either built or leveraged, and their limitations. This includes keeping documentation up to date, not just for compliance but to foster internal understanding and external trust.
Just as important is assigning clear ownership. Each phase of the AI Lifecycle should have clearly defined roles and responsibilities. When teams know who is accountable for a system’s outcomes, it becomes easier to identify problems, make improvements, and ensure AI is being used in a way that aligns with organizational values.
Operational Integration and Monitoring
Effective AI governance requires change management practices that are built into day-to-day development and are not added after the fact. This means establishing clear protocols for how models are introduced, updated, and retired over time. Version control, impact assessments, and approval workflows help ensure that changes to data, code, or behavior are intentional, traceable, and aligned with the right goals.
Once AI systems are live and begin to evolve, so should the oversight. Ongoing monitoring is crucial for catching issues like hallucinations or unintended use. Detection alone isn’t enough–organizations need predefined response plans and escalation paths to address problems quickly and responsibly.
Establish an AI Governance Framework with Expert Guidance
Quisitive’s Generative AI Security & Governance Assessment will help you understand ethical and responsible AI principles and establish critical AI policies and governance procedures.